What is The Main Goal of Generative AI
Discover the main goal of Generative AI: to enhance human creativity through automated content generation. Learn about its key objectives, applications, and the potential impact on industries like media, healthcare, and finance.
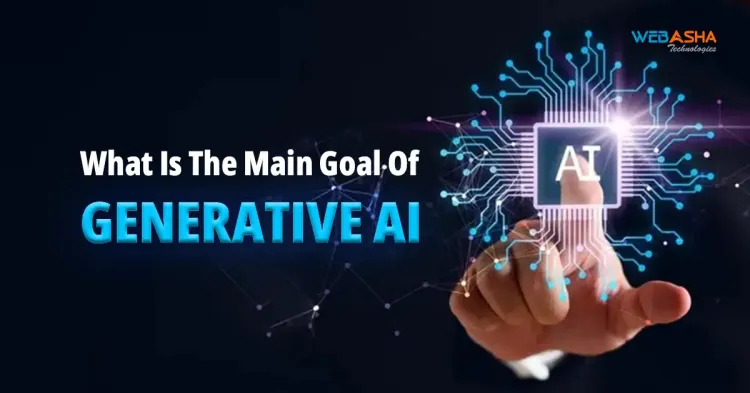
Generative AI, a rapidly evolving field within artificial intelligence, is designed to create new content by learning patterns from existing data. Unlike traditional AI models that follow pre-defined rules or simply make predictions, generative AI goes a step further by generating text, images, music, code, and more, often with an impressive level of creativity and accuracy. But what exactly is the main goal of generative AI? Let’s delve into its core objectives and understand its transformative potential.
Understanding of Generative AI?
Generative AI is a subset of artificial intelligence that focuses on creating new content—such as text, images, music, videos, and even code—by learning patterns from existing data. Unlike traditional AI, which primarily analyzes data or makes predictions, generative AI is designed to generate new outputs that are often indistinguishable from human-created content. This capability is achieved through advanced algorithms and neural networks, such as Generative Adversarial Networks (GANs) and Transformer models like GPT (Generative Pre-trained Transformer).
The Core Goal of Generative AI
The main goal of generative AI is to replicate and enhance human creativity by autonomously generating content that is original, high-quality, and contextually relevant. This involves using complex algorithms and neural networks, such as Generative Adversarial Networks (GANs) and Transformer models like GPT (Generative Pre-trained Transformer), to learn from large datasets and produce outputs that closely mimic human-like responses and creativity.
Key Objectives of Generative AI
-
Content Generation and Automation: Generative AI aims to automate the creation of various types of content, from text and images to videos and music. This reduces the time and effort required for human creators and allows for the production of vast amounts of content at scale. For example, AI can generate personalized marketing content, write code, or even compose music, making it a valuable tool across industries.
-
Enhancing Creativity and Innovation: By providing tools that can generate new ideas and solutions, generative AI fosters innovation. It assists creators in brainstorming and exploring novel concepts by offering unique suggestions or prototypes that may not have been conceived through human effort alone.
-
Improving User Experience: Generative AI enhances user interactions by creating personalized experiences. For instance, in customer service, AI can generate tailored responses that closely match the user's needs, providing a more engaging and efficient service.
-
Cost Efficiency and Scalability: One of the practical goals of generative AI is to reduce costs and increase scalability in content production. By automating tasks that would traditionally require significant time and resources, businesses can operate more efficiently and at a lower cost.
-
Learning and Adaptation: Generative AI continuously learns from new data, adapting its outputs to remain relevant and accurate. This goal is crucial for applications like real-time language translation, personalized recommendations, and adaptive gaming experiences, where the AI must evolve with user interactions.
How Generative AI Works
Generative AI relies on complex neural networks that include:
-
Generative Adversarial Networks (GANs): GANs consist of two neural networks—the generator and the discriminator—that work against each other. The generator creates new content, while the discriminator evaluates its authenticity. This competition improves the quality of the generated content over time.
-
Transformer Models: Models like GPT use deep learning techniques to generate human-like text based on input prompts. They are trained on large datasets and can produce coherent and contextually relevant outputs across various applications, from writing articles to answering questions.
Ethical and Practical Considerations
While generative AI offers immense potential, it also poses ethical challenges, such as the creation of deepfakes or other misleading content. There are concerns about intellectual property rights, data privacy, and the potential for AI-generated outputs to spread misinformation. Therefore, responsible use and regulation are crucial as the technology continues to develop.
Applications of Generative AI
Generative AI has diverse applications across multiple industries, driving innovation and efficiency through automated content creation and data analysis. Here are some key use cases across various sectors:
1. Media and Entertainment:
Content Creation: Generates articles, scripts, music, and videos, automating content production and enhancing creativity.
Visual Effects: Creates realistic images and animations for movies and games, reducing costs and time.
2. Healthcare:
Medical Imaging: Enhances images for better diagnosis, generates synthetic data for training models without privacy concerns.
Drug Discovery: Simulates molecular structures and predicts the efficacy of new drugs, speeding up the research process.
3. Marketing and Advertising:
Personalized Marketing: Crafts customized ads, social media content, and emails tailored to specific audiences, boosting engagement.
Brand Design: Generates logos, graphics, and visual content that aligns with brand identity.
4. Finance:
Fraud Detection: Analyzes patterns in financial data to detect anomalies and prevent fraud.
Algorithmic Trading: Creates predictive models to inform trading strategies and optimize investment decisions.
5. Manufacturing:
Product Design: Generates innovative product designs and prototypes using AI-driven simulations and optimizations.
Quality Control: Uses generative models to predict defects and improve production processes.
6. Retail:
Virtual Try-Ons: Creates virtual models of clothing or accessories, allowing customers to see how items would look before purchase.
Inventory Optimization: Predicts demand and optimizes inventory levels, reducing waste and costs.
7. Education:
Content Development: Generates educational materials, quizzes, and personalized learning content.
Tutoring and Assistance: Provides AI-driven tutoring, offering personalized help to students based on their learning pace.
8. Art and Design:
Creative Assistance: Assists artists and designers in generating new ideas, artwork, or design concepts.
Digital Art Creation: Produces original digital artworks based on specific themes or styles.
9. Customer Service:
Chatbots and Virtual Assistants: Provides 24/7 customer support through AI-driven chatbots that can handle common queries and issues.
Personalized Responses: Generates customized responses to enhance customer interactions and satisfaction.
10. Architecture and Real Estate:
Virtual Staging: Creates virtual home stagings to help buyers visualize properties.
Design Optimization: Generates architectural designs and layout options based on specific criteria.
Generative AI is reshaping industries by automating tasks, enhancing creativity, and delivering personalized experiences. As the technology continues to evolve, its applications will only expand, offering even more innovative solutions across various fields.
Generative AI History
Generative AI's history began in the 1950s with early AI concepts and evolved significantly in the 2010s with the development of deep learning techniques. The introduction of Generative Adversarial Networks (GANs) in 2014 by Ian Goodfellow marked a major milestone, allowing AI to generate realistic images. Later, advancements in Transformer models like GPT by OpenAI further revolutionized the field, enabling sophisticated text generation. Today, generative AI continues to expand, influencing various industries by automating and enhancing creative tasks.
Generative AI’s Place in Modern Technology
Generative AI is integral to modern technology, automating content creation in media, personalizing user experiences in marketing, and aiding design in art and healthcare. It enhances productivity, creativity, and innovation across industries, making it a key driver of digital transformation.
Generative AI has become a cornerstone of modern technology, influencing a wide range of industries with its ability to create content that closely mimics human creativity. Its place in modern tech is defined by its innovative applications in areas like:
Content Creation: Generative AI automates the production of text, images, music, and videos, enhancing productivity in media, marketing, and entertainment.
Personalization: It powers recommendation systems, personalized marketing, and customer service chatbots, offering tailored user experiences.
Design and Art: AI tools assist in generating novel designs and art, pushing creative boundaries in fashion, graphic design, and digital art.
Healthcare: In healthcare, generative AI creates synthetic data for research, aids in drug discovery, and generates personalized treatment plans.
Software Development: AI generates code snippets and even full applications, accelerating software development processes.
Generative AI’s integration into modern technology is driving efficiency, creativity, and innovation, making it a pivotal force in the digital transformation of industries. As the technology evolves, its impact will likely continue to grow, reshaping how we interact with and leverage digital content.
What are Dall-E and ChatGPT?
DALL-E and ChatGPT are AI models developed by OpenAI that serve different purposes within the realm of generative AI.
DALL-E:
DALL-E is an image-generating AI model that creates images from text descriptions. It combines natural language processing and computer vision, allowing users to input prompts and receive unique images that match the description. For example, it can generate images of fantastical concepts, like "a cat wearing a spacesuit," blending creativity with detailed, realistic visuals. DALL-E showcases the potential of AI in creative fields such as design, marketing, and digital art.
ChatGPT:
ChatGPT is a conversational AI model based on the GPT (Generative Pre-trained Transformer) architecture. It generates human-like text based on user input, making it useful for tasks like answering questions, drafting emails, writing content, and simulating conversations. ChatGPT excels in understanding and generating natural language, making it a powerful tool for customer service, education, content creation, and more.
Both DALL-E and ChatGPT demonstrate the versatility of AI in generating creative outputs, revolutionizing how we interact with and use technology in everyday applications.
Benefits and Limitations of Generative AI
Benefits of Generative AI
-
Enhanced Creativity: Generates new ideas, designs, and content that push the boundaries of traditional creativity, aiding artists, designers, and content creators.
-
Efficiency and Automation: Automates repetitive tasks such as content creation and data analysis, saving time and reducing labor costs.
-
Personalization: Provides tailored experiences and content, such as customized marketing messages and product recommendations, improving user engagement and satisfaction.
-
Innovation Acceleration: Speeds up product development, drug discovery, and research by generating simulations, prototypes, and predictions.
-
Cost Reduction: Reduces the need for extensive human input and resources in creating content or conducting research, lowering operational costs.
-
Scalability: Enables the production of large volumes of content or data-driven insights quickly, supporting scalability in various applications.
Limitations of Generative AI
-
Quality Variability: The quality of generated content can vary, with some outputs lacking coherence, accuracy, or originality compared to human-created content.
-
Data Dependency: Requires large and diverse datasets for training, which can be costly and time-consuming to compile and may include inherent biases.
-
Context Understanding: May struggle with understanding nuanced context or generating content that fully aligns with specific human values or intentions.
-
Overfitting Risk: Models might overfit to training data, leading to outputs that are too similar to existing content or fail to generalize well.
-
Resource Intensive: Training and running generative AI models can be computationally expensive, requiring significant hardware and energy resources.
Challenges and Ethical Considerations
While the goals of generative AI are ambitious and transformative, they also come with challenges and ethical considerations. The potential for misuse, such as creating misleading information or deepfakes, requires careful regulation and ethical guidelines. Moreover, ensuring that AI-generated content does not infringe on existing copyrights or cultural sensitivities is crucial.
-
Ethical Concerns: Raises issues related to the creation of deepfakes, misinformation, and other potentially harmful or misleading content.
-
Bias and Fairness: Can perpetuate or amplify biases present in training data, leading to biased or unfair outcomes in generated content.
-
Intellectual Property: Challenges related to copyright and ownership of AI-generated content, especially when it closely resembles existing works.
-
Misuse Potential: Risks of misuse in generating fraudulent content, propaganda, or other malicious uses that can have societal or security impacts.
-
Regulation and Control: The need for effective regulation and oversight to ensure responsible use and mitigate negative consequences of generative AI technologies.
-
Privacy Issues: Concerns about the use of personal data in training models, potentially leading to privacy violations or unauthorized data usage.
Generative AI offers significant benefits, including enhanced creativity and efficiency, but also comes with limitations and challenges that need to be addressed through careful design, ethical considerations, and regulatory measures. Balancing its potential with responsible use is key to maximizing its positive impact.
Generative AI vs. Traditional AI
Aspect | Generative AI | Traditional AI |
---|---|---|
Purpose | Creation of new content (e.g., text, images, music) | Data analysis and decision making |
Functionality | Uses models like GANs or Transformers to generate novel content | Uses algorithms for classification, regression, or predictions |
Output | Produces original outputs resembling human creativity | Provides insights, classifications, or predictions based on data |
Applications | Art and design, content creation, healthcare | Fraud detection, customer service, healthcare diagnostics |
Training Data | Requires diverse and extensive datasets | Utilizes targeted and specific datasets |
Challenges | Quality control, ethical concerns (e.g., misuse) | Data bias, model interpretability |
This table highlights the core differences between Generative AI and Traditional AI in terms of purpose, functionality, output, applications, training data, and challenges.
Future of Generative AI: Trends and Predictions
The future of Generative AI is set to be transformative, with several key trends and predictions shaping its evolution:
-
Wider Integration: Generative AI will increasingly become a part of everyday tools and applications, enhancing productivity and creativity in areas like content creation, marketing, and design.
-
Enhanced Creativity: Advances in technology will lead to even more creative and realistic outputs. AI will be able to generate more sophisticated content, pushing the boundaries of what’s possible.
-
Personalization: Generative AI will provide highly personalized experiences by tailoring recommendations, advertisements, and interactions to individual preferences and behaviors.
-
Ethical and Regulatory Developments: As the technology becomes more prevalent, there will be a stronger focus on establishing ethical guidelines and regulatory frameworks to address issues such as misuse, bias, and privacy concerns.
-
Diverse Applications: AI will find applications across various sectors, including healthcare, finance, education, and entertainment, driving innovation and efficiency in these fields.
-
Improved Collaboration Tools: Tools for collaboration and content creation will be enhanced with generative AI, making teamwork and project management more dynamic and interactive.
-
Advancements in Research: Generative AI will play a significant role in scientific research, helping to generate new hypotheses, simulate experiments, and contribute to academic publications.
-
Better Human-AI Collaboration: The focus will shift to improving how humans and AI work together, with AI augmenting human creativity and decision-making rather than replacing it.
-
Real-Time Capabilities: Advances will enable AI to generate content in real-time, enhancing experiences in areas such as gaming, virtual reality, and live media.
-
AI for Social Impact: Generative AI will increasingly be used for social good, including applications in disaster response, educational tools, and environmental sustainability.
In summary, Generative AI is poised to revolutionize many aspects of our lives by driving creativity, personalization, and innovation while also addressing important ethical and social issues.
Future Trends and Predictions
Trend/Prediction | Description |
---|---|
More Integration | Generative AI will be used more in everyday tools, making tasks like content creation and marketing easier. |
Better Creativity and Quality | Future improvements will make AI-generated content even more creative and realistic. |
Personalized Experiences | AI will create tailored recommendations and ads just for you, based on your preferences. |
Ethics and Regulations | There will be new rules and guidelines to handle issues like misuse, bias, and privacy concerns. |
Wide Use Across Fields | Generative AI will be applied in many areas, including healthcare, finance, and education, to drive progress. |
Improved Collaboration Tools | Work tools will be enhanced with AI, making team projects and content creation more dynamic. |
AI in Research | AI will help in research by suggesting ideas, running simulations, and contributing to studies. |
Better Human-AI Teamwork | AI will work alongside humans to boost creativity rather than replace it. |
Real-Time Generation | Advances will allow AI to create content instantly, improving experiences in games, virtual reality, and live media. |
AI for Social Good | AI will be used to address social issues, including disaster response, education, and environmental efforts. |
The Ethical Implications of Generative AI
The rise of Generative AI brings with it a range of ethical implications that need careful consideration. Here are some of the key ethical issues associated with this technology:
1. Misinformation and Deepfakes
Generative AI can create highly convincing fake content, such as deepfakes, which can be used to spread misinformation or deceive people. This poses risks to public trust, personal privacy, and can influence elections or cause reputational harm.
2. Bias and Fairness
AI models are trained on existing data, which can include biases. If not managed properly, Generative AI can perpetuate or amplify these biases, leading to unfair or discriminatory outcomes in the content it produces or the decisions it influences.
3. Intellectual Property and Copyright
Generative AI can produce content that closely resembles existing works, raising questions about intellectual property rights. Issues arise over who owns the rights to AI-generated content and how to handle potential copyright infringement.
4. Privacy Concerns
Generative AI often relies on large datasets, which may include personal information. There are concerns about how this data is collected, stored, and used, and how to ensure that individual privacy is protected.
5. Accountability and Responsibility
Determining who is accountable for the actions and outputs of Generative AI can be challenging. If AI generates harmful or unethical content, it's crucial to establish who is responsible—the developers, users, or the AI itself.
6. Security Risks
Generative AI could be used to create sophisticated phishing attacks, scams, or other malicious activities. Ensuring that the technology is used responsibly and does not pose security threats is an ongoing challenge.
7. Impact on Employment
As Generative AI becomes more advanced, there is concern about its impact on jobs, particularly in creative fields. While it can enhance productivity, it might also lead to job displacement and changes in the nature of work.
8. Authenticity and Trust
The ability of Generative AI to produce highly realistic content can blur the line between what is real and what is artificial. This can erode trust in digital media and make it harder for people to discern authentic content from generated content.
9. Ethical Use of AI-generated Content
Deciding how and when to use AI-generated content ethically is crucial. There must be guidelines to prevent misuse and ensure that the content is used responsibly and aligns with ethical standards.
10. Transparency and Explainability
Generative AI models can be complex and opaque, making it difficult to understand how they produce their outputs. Promoting transparency and explainability is important for building trust and ensuring that the technology is used ethically.
In summary, while Generative AI offers exciting possibilities, it also presents significant ethical challenges. Addressing these issues requires a thoughtful approach, including the development of ethical guidelines, regulatory measures, and ongoing dialogue among technologists, policymakers, and the public.
How to Get Started with Generative AI
Getting started with Generative AI involves understanding the basics, setting up the necessary tools, and exploring practical applications. Here’s a step-by-step guide to help you begin:
1. Understand the Basics
Learn the Fundamentals: Familiarize yourself with core concepts of AI and machine learning, including neural networks, deep learning, and how Generative AI works. Resources like online courses, tutorials, and articles can be helpful.
Explore Key Models: Understand popular Generative AI models such as Generative Adversarial Networks (GANs), Variational Autoencoders (VAEs), and Transformer-based models like GPT (Generative Pre-trained Transformer).
2. Choose Your Focus Area
Determine Your Interest: Decide which area of Generative AI interests you most, such as image generation, text generation, or music composition. This will help you focus your learning and development efforts.
3. Acquire the Necessary Skills
Programming Knowledge: Gain proficiency in programming languages commonly used in AI, such as Python. Libraries and frameworks like TensorFlow, PyTorch, and Keras are essential for developing and experimenting with AI models.
Mathematics and Statistics: Brush up on mathematical concepts like linear algebra, calculus, and probability, which are foundational for understanding AI algorithms and models.
4. Set Up Your Environment
Choose a Development Environment: Install and set up tools for AI development. Jupyter Notebooks, Google Colab, and integrated development environments (IDEs) like PyCharm or VS Code are popular choices.
Install AI Libraries: Set up necessary libraries and frameworks for Generative AI. Libraries such as TensorFlow, PyTorch, and Hugging Face’s Transformers are commonly used.
5. Start with Pre-trained Models
Use Pre-trained Models: Begin by experimenting with pre-trained models available from sources like Hugging Face’s Model Hub or TensorFlow Hub. These models can help you understand how Generative AI works and allow you to fine-tune them for specific tasks.
6. Work on Projects
Build Simple Projects: Start with small projects to apply what you’ve learned. Examples include generating text with GPT-3, creating images with GANs, or composing music with AI models.
Participate in Competitions: Join AI competitions on platforms like Kaggle to tackle real-world problems and gain hands-on experience.
7. Explore Resources and Communities
Read Research Papers: Stay updated with the latest advancements in Generative AI by reading research papers and articles from conferences like NeurIPS, ICML, and CVPR.
Join Communities: Engage with online communities and forums like Reddit’s r/MachineLearning, AI-specific Slack groups, or local meetups to network with others and get support.
8. Ethical Considerations
Understand Ethics: Familiarize yourself with the ethical implications of Generative AI and consider how to use the technology responsibly. Ensure you’re aware of issues related to bias, privacy, and the impact of your work.
9. Keep Learning
Stay Updated: The field of Generative AI is rapidly evolving. Continuously seek out new resources, tutorials, and research to keep your skills and knowledge up to date.
By following these steps, you can effectively get started with Generative AI and begin exploring its potential.
Conclusion
The primary goal of generative AI is to extend the boundaries of what machines can create, replicating and even enhancing human creativity. By automating content generation, enhancing innovation, and improving user experiences, generative AI is set to play a pivotal role in numerous industries. However, as its applications grow, it’s essential to balance its capabilities with responsible use to harness its full potential for positive impact.
Generative AI isn't just about replicating what humans can do; it's about expanding those possibilities, pushing the frontiers of creativity, and opening new avenues for technological and artistic exploration. As this technology continues to evolve, its goals will likely expand, further blending the line between human and machine creativity.
Generative AI represents a significant leap forward in artificial intelligence, with the ability to create content that rivals human creativity. Its applications are broad and continue to grow, making it an invaluable tool across various industries. As we embrace this technology, it’s important to balance innovation with ethical considerations to fully harness the benefits of generative AI while mitigating potential risks.
FAQs
1. What is Generative AI?
Generative AI refers to a branch of artificial intelligence that focuses on creating new content, such as text, images, music, or code, by learning from existing data. It uses models like Generative Adversarial Networks (GANs) and Transformer models (e.g., GPT) to generate outputs that mimic human creativity.
2. What is the main goal of Generative AI?
The main goal of Generative AI is to replicate and enhance human creativity by autonomously generating original, high-quality, and contextually relevant content. It aims to automate content creation, foster innovation, improve user experiences, and increase efficiency across various industries.
3. How does Generative AI work?
Generative AI works by using deep learning algorithms that learn patterns from large datasets. Models like GANs consist of two networks—a generator and a discriminator—that work together to create realistic outputs. Transformer models, like GPT, use vast amounts of data to predict and generate text or other content based on the input they receive.
4. What are the applications of Generative AI?
Generative AI is used in various fields, including entertainment (e.g., creating deepfakes, scripts), healthcare (e.g., generating synthetic data for training models), art and design (e.g., creating new artwork), and finance (e.g., generating predictive models). Its applications are diverse and continuously expanding.
5. What are some key challenges of Generative AI?
Challenges of Generative AI include ethical concerns like the potential misuse of deepfakes or misinformation, ensuring content quality and accuracy, managing biases in generated outputs, and respecting intellectual property rights. There are also technical challenges related to the complexity of model training and the computational resources required.
6. How does Generative AI improve user experience?
Generative AI enhances user experience by personalizing content, automating customer service interactions, and generating tailored recommendations. For example, AI can create personalized marketing messages or chat responses that improve engagement and satisfaction.
7. What ethical considerations are associated with Generative AI?
Ethical considerations include the risk of generating harmful or misleading content, the potential for copyright infringement, and privacy concerns when creating synthetic data. Ensuring transparency, accountability, and responsible use of Generative AI is crucial to addressing these issues.
8. How is Generative AI different from traditional AI?
Traditional AI often focuses on classification, prediction, and decision-making tasks based on predefined rules or data patterns. Generative AI, on the other hand, is designed to create new, original content by understanding and replicating the nuances of human creativity.
9. Can Generative AI replace human creativity?
Generative AI is not meant to replace human creativity but rather to enhance it. While it can automate certain creative tasks, it still relies on human input, guidance, and oversight to ensure that the generated content aligns with desired goals and ethical standards.
10. What is the future of Generative AI?
The future of Generative AI is promising, with advancements expected in fields like personalized content creation, virtual reality, and advanced simulations. As technology continues to evolve, Generative AI will likely become more sophisticated, making it an integral part of creative and business processes.