What is Agentic AI ? Agentic AI vs. Generative AI - Key Differences, Use Cases & Future Explained
Discover the core differences between Agentic AI and Generative AI. Learn how each works, their real-world applications, strengths, challenges, and what the future holds for AI-driven automation and creativity. Agentic AI, Generative AI, Agentic AI vs Generative AI, AI types comparison, AutoGPT, BabyAGI, Devin AI, ChatGPT, AI automation, AI agents, future of AI, generative AI examples, agentic AI use cases, AI decision-making, AI planning and memory, LLMs, artificial intelligence trends
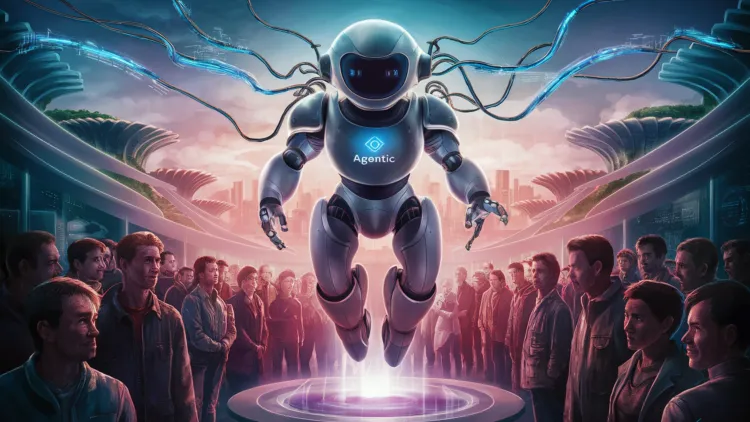
Introduction
Artificial Intelligence (AI) refers to the ability of machines to mimic human intelligence in order to perform tasks such as learning, reasoning, problem-solving, and understanding language. Over the past few years, AI has evolved at a breathtaking pace. What started as simple rule-based systems has now grown into sophisticated technologies capable of producing human-like conversations, creating art, writing code, and even making complex decisions.
With this rapid evolution, different types of AI have emerged—each serving a unique purpose. Two of the most talked-about categories today are Generative AI and Agentic AI. While both fall under the AI umbrella, they function very differently. Understanding their distinctions is essential not just for developers and tech professionals, but also for businesses, policymakers, and everyday users who rely on AI tools in various ways.
This article aims to explain the core concepts of Generative AI and Agentic AI, compare how they work, highlight their strengths and limitations, and explore their real-world applications. By the end, you’ll have a clear picture of how these two AI approaches are shaping the future—and why it matters.
What is Generative AI?
Generative AI is a type of artificial intelligence designed to create new content. It doesn't just process data—it produces something new from it. Whether it’s writing an article, generating an image, composing music, or suggesting code, generative AI can craft outputs that resemble human creativity.
How Does Generative AI Work?
At the core of most modern generative AI systems are transformer models, a type of deep learning architecture. These models are trained on massive datasets—such as books, websites, images, or programming languages—to recognize patterns and relationships within the data.
When you give a prompt (like a sentence or an image description), the model uses what it has learned to generate an appropriate response or output. It doesn't “understand” the content the way humans do, but it uses probabilities to predict what comes next, based on training.
For example:
-
If you ask ChatGPT to write a blog post, it predicts and generates text based on patterns found in similar articles.
-
If you use DALL·E to generate an image of “a robot walking a dog in space,” it visualizes this concept based on millions of prior image-text pairs.
Common Examples of Generative AI
-
ChatGPT – Conversational AI that can answer questions, write essays, or generate ideas.
-
Midjourney – A tool that turns text prompts into detailed digital artwork.
-
DALL·E – An image generator developed by OpenAI that transforms textual descriptions into images.
-
GitHub Copilot – An AI-powered coding assistant that helps developers write code more efficiently.
Strengths and Limitations
Strengths:
-
Excellent for creative tasks like writing, designing, coding, and ideation.
-
Fast, scalable, and capable of producing a wide range of content on demand.
-
Can boost productivity in various industries, from marketing to software development.
Limitations:
-
Lacks autonomy—it requires human input to function.
-
Doesn’t truly “understand” context or emotions; responses are based on statistical patterns, not reasoning.
-
May generate incorrect, biased, or nonsensical content if not carefully managed.
In essence, generative AI is like a very talented assistant: it’s great at producing content when asked, but it won’t take initiative or make decisions without your direction.
What is Agentic AI?
Agentic AI represents the next step in artificial intelligence, where systems are not just passive responders but active participants. These AI agents are built with the ability to set goals, make decisions, plan actions, and carry out tasks autonomously—often without constant human supervision.
Unlike generative AI, which waits for a prompt and gives back a result, agentic AI behaves more like a digital agent. It takes initiative, can break down complex tasks into steps, use external tools, and even adjust its approach based on feedback.
Core Components of Agentic AI
To function independently, agentic AI systems are made up of several advanced features:
-
Planning – Breaking down a goal into smaller steps and creating a logical path to achieve it.
-
Reasoning – Making decisions based on available data, constraints, and objectives.
-
Memory – Storing context or results from previous interactions for smarter future behavior.
-
Tool Use – Accessing external APIs, web browsers, or plugins to complete tasks.
-
Self-Correction – Evaluating its own outputs and revising actions when errors occur.
Real-World Analogy
Imagine hiring a virtual assistant. A generative AI would give you suggestions or write an email when you ask. An agentic AI, on the other hand, would:
-
Draft the email,
-
Look up contacts,
-
Send the message,
-
Schedule a follow-up meeting,
-
And remind you before the event — all by itself.
Popular Examples of Agentic AI
-
AutoGPT – An autonomous AI agent that can plan and execute tasks across multiple steps using GPT models.
-
BabyAGI – A lightweight AI system capable of generating, prioritizing, and executing tasks based on objectives.
-
OpenAI's GPT Agents with Tools – Enhanced GPT-4 agents that use browsing, code execution, and file handling tools.
-
Devin AI – An AI software engineer capable of completing entire coding projects autonomously.
Key Differences Between Agentic AI and Generative AI
Here’s a side-by-side breakdown of how Agentic AI and Generative AI differ fundamentally:
Feature | Generative AI | Agentic AI |
---|---|---|
Purpose | Content creation (text, image, code, etc.) | Goal-driven task completion |
Input/Output | One prompt → One response | Multi-step reasoning and continuous output |
Memory | Usually stateless | Often uses short- and long-term memory |
Autonomy | Reactive (waits for user input) | Proactive (acts on its own to fulfill tasks) |
Use Cases | Text generation, chatbots, image creation | Workflow automation, research bots, task agents |
Generative AI is like a skilled artist or writer waiting for instruction. Agentic AI is more like a project manager who can not only create but also organize, plan, and deliver results independently.
Use Cases and Applications
A. Generative AI Use Cases
Generative AI is already revolutionizing creative industries and everyday productivity. Common use cases include:
-
Blog/Content Creation – Drafting SEO articles, emails, and marketing copy.
-
Art and Design – Producing digital art, logos, and creative visuals from text prompts.
-
Chatbots and Virtual Assistants – Handling customer queries, generating responses, and simulating human conversation.
B. Agentic AI Use Cases
Agentic AI shines in situations where long-term planning and task management are needed. Use cases include:
-
Autonomous Coding Agents – Writing, testing, and debugging code based on project goals.
-
Market Analysis Bots – Researching trends, analyzing competitor data, and reporting insights.
-
Personal Task Agents – Handling to-do lists, scheduling appointments, and automating repetitive tasks.
-
Enterprise Workflow Automation – Managing and automating complex operations like HR onboarding or IT ticketing without human intervention.
Challenges and Risks
While both Generative AI and Agentic AI offer powerful capabilities, they also come with serious challenges that developers, users, and society at large must address. Understanding these risks is essential to ensure that AI technologies are used responsibly and ethically.
A. Challenges and Risks of Generative AI
Generative AI has gained widespread popularity due to its ability to create human-like content across various formats. However, its growing influence also brings some major concerns:
1. Misinformation and Fake Content
Generative AI can easily produce realistic-looking text, images, and videos. This opens the door to:
-
Deepfakes – Fake images or videos of public figures.
-
False news articles – Misleading content that can go viral.
-
Fake reviews or product descriptions – Manipulating consumer opinion.
Since the AI has no way of knowing what's true or false, it can unintentionally spread misinformation.
2. Bias and Discrimination
Generative models are trained on massive datasets from the internet—which includes biased or offensive content. As a result, the AI can sometimes:
-
Produce biased language or stereotypes.
-
Exclude certain groups in image generation or text responses.
-
Reinforce harmful narratives unintentionally.
Ensuring fairness and inclusivity in AI output remains a big challenge.
3. Copyright and Ownership Issues
Generative AI often replicates styles or patterns it has seen in training data. This raises questions like:
-
Who owns the output—user or model creator?
-
Did the AI unintentionally plagiarize someone’s copyrighted work?
-
Is it fair use or infringement?
These are ongoing legal and ethical debates in the AI community.
B. Challenges and Risks of Agentic AI
Agentic AI brings even more complexity due to its ability to make decisions and take autonomous actions. While this autonomy is powerful, it also introduces new layers of risk:
1. Safety and Control
Unlike generative AI, which only responds to prompts, agentic AI takes initiative. That makes it harder to control. For example:
-
An agentic AI tasked with “maximize engagement” might take extreme steps, like posting sensational content.
-
If given vague or overly ambitious goals, it might behave in unexpected or unsafe ways.
Goal alignment and safety constraints are crucial, yet difficult to perfect.
2. Unpredictable Behavior and Hallucinations
Agentic AI systems often depend on large language models (LLMs), which can sometimes generate false or illogical outputs—known as hallucinations.
In simple chatbots, this is harmless. But in agentic systems that chain decisions together, one bad assumption can:
-
Spiral into a completely flawed execution plan.
-
Cause wasted resources, misinformation, or even real-world harm.
3. Security and Misuse Potential
Agentic AI has the power to automate entire workflows—this makes it a potential tool for malicious purposes, such as:
-
Phishing and scam automation – Writing convincing emails and sending them out autonomously.
-
Vulnerability scanning bots – Searching websites or systems for security holes.
-
Mass content generation for spam or propaganda.
Without proper oversight, agentic AI could become a powerful tool in the hands of bad actors.
The Future of Agentic and Generative AI
As artificial intelligence continues to evolve rapidly, the future lies in how we blend the creative capabilities of generative AI with the decision-making power of agentic AI. The next frontier will not be about choosing between the two—but about how they work together to create more intelligent, autonomous systems that can assist in real-world applications.
1. Will Agentic AI Become the Default Interface for Interacting with AI?
Yes, and here’s why.**
The current model of interacting with AI—where users type a question and receive a response—is useful but limited. It still requires users to interpret and act on the information themselves.
Agentic AI, however, changes that dynamic. Instead of simply providing answers, it can:
-
Interpret user intent,
-
Plan a sequence of actions,
-
Execute those tasks across multiple platforms.
Imagine an AI that not only helps you write an email but also schedules a meeting, books a venue, and sends invites—without needing further prompts. This level of interaction is more natural, productive, and aligned with how people want to use AI in daily life.
As these systems improve, agentic AI is likely to become the standard way users interact with AI—not just for tech-savvy users but for everyday consumers, businesses, and educators.
2. Integration of Generative AI Within Agentic Systems
Agentic AI doesn’t replace generative AI—it builds on it.
In fact, generative AI is a core engine within many agentic systems. These systems use generative models to:
-
Write code,
-
Draft emails,
-
Compose reports,
-
Generate responses in multi-step tasks.
An agentic AI system often relies on a generative model (like GPT-4 or Claude) to do the “thinking” and language generation, while the agentic framework handles orchestration, decision-making, and memory.
This integration creates more powerful hybrid systems—capable of both creativity and autonomy.
3. Role in Enterprise, Research, and Personal Productivity
The practical uses of both generative and agentic AI are already transforming key areas:
In Enterprise
-
Automated business processes: From customer support to financial reporting.
-
AI assistants for teams: Handling documentation, scheduling, internal communication, and project management.
-
Data-driven decision-making: Automating market research, competitive analysis, and strategic planning.
In Research
-
Scientific exploration: Agentic systems can autonomously scan papers, generate hypotheses, and even run simulations.
-
Coding and analysis: Tools like Devin AI are already performing software development and debugging autonomously.
In Personal Productivity
-
Smart task assistants: AI that handles to-do lists, personal finances, travel planning, and more.
-
Learning companions: Adaptive agents that personalize your education and help you learn more effectively.
As the technology matures, these applications will become more refined, accessible, and tailored to individual needs.
4. Ethical Considerations and Governance
With great power comes great responsibility. As we adopt more autonomous systems, ethical concerns become increasingly important:
-
Accountability: Who is responsible when an AI agent makes a mistake or causes harm?
-
Transparency: Users must understand how decisions are made and which data is used.
-
Bias and fairness: Ensuring that AI agents don’t reinforce harmful stereotypes or discriminate in task execution.
-
Security and misuse: Preventing these systems from being used for malicious purposes like disinformation or hacking.
Governments, developers, and organizations will need clear regulations, ethical guidelines, and audit mechanisms to ensure these powerful technologies benefit society without causing harm.
Frequently Asked Questions (FAQs)
1. What is Agentic AI?
Agentic AI refers to artificial intelligence systems that can autonomously plan, make decisions, and take actions to achieve specific goals. Unlike generative AI, which responds to a prompt, agentic AI operates proactively, often without continuous human input.
2. What is Generative AI?
Generative AI creates new content such as text, images, music, and code using models trained on large datasets. Examples include ChatGPT, DALL·E, and Copilot, which generate outputs based on input prompts.
3. How is Agentic AI different from Generative AI?
Generative AI produces content based on a single prompt. Agentic AI takes that capability further by using reasoning, memory, and planning to complete multi-step tasks, often with minimal user involvement.
4. Can Agentic AI use Generative AI models?
Yes, most agentic AI systems rely on generative models like GPT-4 to perform tasks that require creativity or natural language understanding. The generative model becomes part of the agent's “brain.”
5. What are some real-world examples of Agentic AI?
Examples include AutoGPT, BabyAGI, OpenAI’s GPT agents with tools, and Devin AI. These agents can write code, automate workflows, or even research topics autonomously.
6. Is Agentic AI better than Generative AI?
Not necessarily. They serve different purposes. Generative AI is ideal for content creation, while Agentic AI excels at completing complex, goal-driven tasks through automation and reasoning.
7. What technologies power Agentic AI?
Agentic AI combines several components: large language models (LLMs), memory modules, reasoning engines, planning frameworks, and tool-use capabilities like APIs or web browsing.
8. Does Agentic AI have memory?
Yes, many agentic AI systems use short- and long-term memory to recall past steps, refine strategies, and track progress over time. Generative AI, in contrast, is usually stateless.
9. What are the risks of Agentic AI?
Key risks include unpredictable behavior, hallucinations during long tasks, potential misuse (e.g., automating scams), and ethical concerns around autonomy and control.
10. What are the risks of Generative AI?
Risks include spreading misinformation, replicating bias from training data, and copyright concerns if it generates content too similar to existing works.
11. What industries can benefit from Agentic AI?
Industries like software development, customer service, market research, and operations management can leverage agentic AI for automation, efficiency, and intelligent decision-making.
12. Can Agentic AI replace human jobs?
Agentic AI may automate certain repetitive or rule-based tasks. However, it’s more likely to augment human capabilities, helping people work faster and focus on high-level decision-making.
13. Is Agentic AI available to the public?
Yes, tools like AutoGPT and Devin AI have open-source or commercial versions. However, they may require technical expertise to set up or customize.
14. How does Agentic AI handle failure or incorrect decisions?
Advanced agentic AI systems may include self-correction mechanisms or allow human oversight. However, failure handling is still an active area of research.
15. Is Generative AI used in everyday applications?
Absolutely. Tools like ChatGPT, Grammarly, Canva (with AI design features), and Google Bard use generative AI to help users write, design, or summarize content.
16. Can I build my own Agentic AI system?
Yes, with frameworks like LangChain, AutoGPT, and OpenAI’s API tools, developers can build agentic workflows. However, it does require knowledge in coding and prompt engineering.
17. Will Agentic AI become the standard way we use AI?
Many experts believe so. As agentic systems become more reliable, they could become the default interface—automating tasks instead of just assisting with them.
18. How are companies using Agentic AI?
Companies are using it for automated coding, intelligent customer support, lead generation, research assistance, and workflow automation in areas like HR, finance, and marketing.
19. How can we ensure Agentic AI remains safe and ethical?
By establishing clear goals, including guardrails, enabling human-in-the-loop review, using audit logs, and following industry standards for responsible AI development.
20. What is the future of Generative and Agentic AI?
The future likely involves deeper integration—where generative AI handles creativity, and agentic AI handles execution. Together, they’ll power next-gen tools in personal productivity, enterprise automation, and advanced research.