How Has Generative AI Affected Security in Cyber Security
Discover how generative AI is transforming cybersecurity with enhanced threat detection, sophisticated attack vectors, and advanced security automation. Learn about the benefits and risks of AI in security, including its impact on phishing, deepfake technology, and evolving threat tactics. Explore mitigation strategies to address AI-specific risks and strengthen your cybersecurity posture.
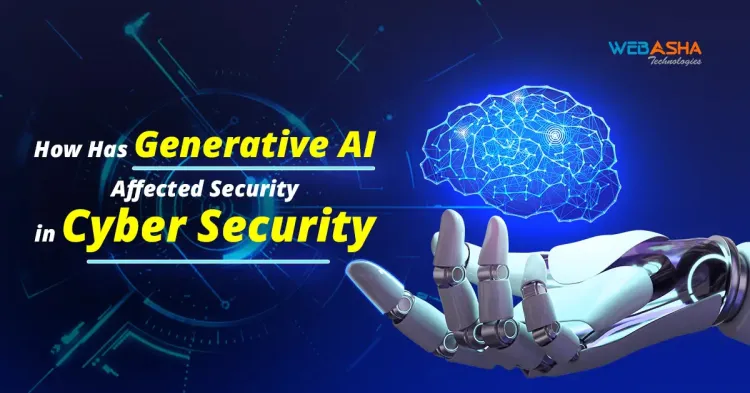
Understanding Generative AI in Cybersecurity
Generative AI, a subset of artificial intelligence, refers to algorithms and models that can generate new content or data based on patterns learned from existing data. In cybersecurity, generative AI plays a significant role in both enhancing defense mechanisms and posing new risks. Here's an in-depth look at how generative AI impacts cybersecurity:
What is Generative AI?
Generative AI involves machine learning models that can create new data, such as images, text, or audio, that closely resembles the data they were trained on. Common types of generative AI include Generative Adversarial Networks (GANs) and Variational Autoencoders (VAEs).
Generative Adversarial Networks (GANs): Consist of two neural networks, a generator and a discriminator, that work against each other to create realistic data.
Variational Autoencoders (VAEs): Use probabilistic models to generate new data based on the distribution of the training data.
Applications of Generative AI in Cybersecurity
Enhanced Threat Detection
- Anomaly Detection: Generative AI models can learn normal network behavior and identify deviations that may indicate potential threats or attacks.
- Predictive Analysis: AI can predict emerging threats by analyzing patterns and trends from historical data.
Advanced Security Tools
- Automated Security Monitoring: AI can generate alerts and reports based on real-time analysis of network traffic and security events.
- Phishing Detection: AI models can create sophisticated algorithms to detect and block phishing attempts by analyzing email content and sender behavior.
Improved Incident Response
- Automated Response Actions: Generative AI can automate responses to detected threats, such as isolating compromised systems or blocking malicious activities.
- Incident Analysis: AI can assist in analyzing security incidents by generating detailed reports and identifying root causes.
Risks and Challenges of Generative AI in Cybersecurity
Sophisticated Cyber Attacks
- Deepfakes: Generative AI can create realistic but fake media, leading to identity theft, misinformation, and social engineering attacks.
- Polymorphic Malware: AI can generate malware that constantly changes its code to evade detection by traditional security measures.
Exploiting AI Vulnerabilities
- Adversarial Attacks: Attackers can use generative AI to create inputs that deceive AI systems, leading to incorrect decisions or classifications.
- Model Inversion: Generative AI can infer sensitive information about the data used to train AI models, potentially exposing confidential information.
Ethical and Privacy Concerns
- Misuse of AI: Generative AI technologies can be misused to create harmful content or conduct malicious activities.
- Privacy Risks: The generation of synthetic data can lead to privacy concerns if it inadvertently reveals sensitive information.
Securing Tomorrow: Generative AI in Cybersecurity
Generative AI is rapidly reshaping the landscape of cybersecurity, offering both groundbreaking advancements and new challenges. As organizations prepare for the future, understanding how generative AI can both enhance and disrupt security measures is crucial. Here’s an in-depth exploration of how generative AI is influencing the field of cybersecurity and how to secure tomorrow with its advancements.
The Role of Generative AI in Cybersecurity
Generative AI encompasses technologies that can produce new data and content by learning from existing datasets. This capability is transforming cybersecurity in several ways:
Advanced Threat Detection
- Anomaly Detection: Generative AI models can establish a baseline of normal network behavior and quickly identify deviations that might signal a security breach.
- Predictive Analytics: By analyzing historical threat data, AI can anticipate future attack vectors and help organizations prepare for emerging threats.
Enhanced Security Measures
- Automated Monitoring: AI-driven systems can continuously analyze network traffic and system activities to detect potential threats in real-time.
- Phishing and Fraud Prevention: AI models can create sophisticated algorithms to detect and block phishing attempts by analyzing patterns in communication and user behavior.
Improved Incident Response
- Automated Incident Handling: Generative AI can automate responses to security incidents, such as isolating affected systems or blocking suspicious activities.
- Post-Incident Analysis: AI can generate detailed reports and provide insights into the root causes of security incidents, aiding in faster recovery and prevention of future attacks.
Emerging Risks and Challenges
While generative AI offers numerous benefits, it also introduces several risks and challenges that organizations must address:
Sophisticated Attack Techniques
- Deepfakes: AI-generated deepfakes can be used for identity theft, misinformation, and sophisticated social engineering attacks.
- Adaptive Malware: Generative AI can create malware that evolves its code to evade traditional detection methods, increasing the difficulty of identifying and neutralizing threats.
Vulnerabilities in AI Systems
- Adversarial Attacks: Attackers may use generative AI to create inputs that deceive AI systems, leading to incorrect threat assessments or security responses.
- Data Leakage: Generative models can potentially infer sensitive information from training data, posing privacy and confidentiality risks.
Ethical and Privacy Concerns
- Misuse of Technology: Generative AI has the potential to be misused for malicious purposes, necessitating strict ethical guidelines and oversight.
- Privacy Risks: The creation of synthetic data raises concerns about the potential for inadvertently exposing personal or confidential information.
Strategies for Securing Tomorrow
To harness the benefits of generative AI while mitigating its risks, organizations should adopt a comprehensive approach:
Implement Robust AI-Driven Security Solutions
- Invest in AI Tools: Deploy advanced AI-based security solutions that enhance threat detection, response, and automation capabilities.
- Continuous Learning: Ensure that AI models are regularly updated and trained on diverse datasets to maintain their effectiveness against evolving threats.
Address AI-Specific Risks
- Ethical Practices: Adhere to ethical guidelines for the development and deployment of generative AI to prevent misuse and ensure responsible use.
- Data Protection: Implement strong data protection measures to safeguard sensitive information and prevent data leakage.
Foster Collaboration and Awareness
- Industry Partnerships: Collaborate with industry peers and participate in information-sharing initiatives to stay informed about AI-driven threats and mitigation strategies.
- Ongoing Education: Provide training for security professionals on the latest developments in generative AI and best practices for managing AI-related risks.
Conclusion
Generative AI is transforming cybersecurity by providing advanced tools for threat detection and response, but it also introduces new risks and challenges. Understanding both the benefits and potential threats of generative AI is essential for developing effective security strategies and maintaining a robust cybersecurity posture.
By staying informed and adopting proactive measures, organizations can harness the power of generative AI to enhance their security while mitigating associated risks.
Generative AI has brought both opportunities and challenges to the field of cybersecurity. On one hand, it has enhanced threat detection, response capabilities, and security automation. On the other hand, it has introduced new risks, such as sophisticated attacks and vulnerabilities related to AI systems. To effectively manage these impacts, organizations must adopt advanced AI-driven security solutions, address AI-specific risks, and foster collaboration within the cybersecurity community.
FAQ's
How has generative AI improved threat detection in cybersecurity?
Generative AI enhances threat detection by automating the analysis of large volumes of security data, identifying anomalies, and detecting patterns indicative of potential threats more quickly and accurately than traditional methods.
What role does generative AI play in phishing detection?
Generative AI improves phishing detection by analyzing email content and identifying patterns that suggest phishing attempts. AI models can generate and recognize sophisticated phishing emails, reducing the likelihood of successful attacks.
How does generative AI contribute to sophisticated cyber attacks?
Generative AI can create advanced deepfakes for identity theft and misinformation, generate personalized phishing attacks, and develop polymorphic malware that changes its code to evade detection by traditional security measures.
What are adversarial attacks in the context of generative AI?
Adversarial attacks involve using generative AI to create inputs that deceive AI systems into making incorrect decisions or classifications, potentially leading to vulnerabilities in security systems.
How can organizations mitigate risks associated with generative AI?
Organizations can mitigate risks by investing in AI-driven security solutions, continuously monitoring for AI-enhanced threats, using robust training data for AI models, and following ethical guidelines for AI deployment.
What is model inversion, and how does it relate to generative AI?
Model inversion involves using generative AI to infer sensitive information about the data used to train AI models, potentially exposing confidential information and creating security risks.
How can generative AI be used in security automation?
Generative AI can automate routine security tasks such as log analysis and alert prioritization, allowing security teams to focus on more complex issues and improving overall response times to threats.
What are deepfakes, and why are they a concern in cybersecurity?
Deepfakes are realistic but fake media generated using AI, which can be used for identity theft, social engineering, and misinformation. They pose a significant risk as they can deceive individuals and organizations, leading to security breaches.
What are the benefits of AI-based security solutions?
AI-based security solutions offer benefits such as enhanced threat detection and response, predictive analytics, and improved automation of routine tasks. They help organizations stay ahead of evolving threats and manage security more effectively.
How can organizations stay informed about AI-driven threats?
Organizations can stay informed by subscribing to threat intelligence feeds, participating in cybersecurity forums, attending conferences, and collaborating with industry peers to share information about emerging AI-driven threats.