How Can Generative AI be Used in Cyber Security
Explore how generative AI is revolutionizing cybersecurity by enhancing threat detection, automating security operations, and improving incident analysis. Learn about its applications in phishing prevention, predictive analytics, and security training. Understand how to address AI-specific risks and ensure responsible use to strengthen your cybersecurity posture and safeguard against emerging threats.
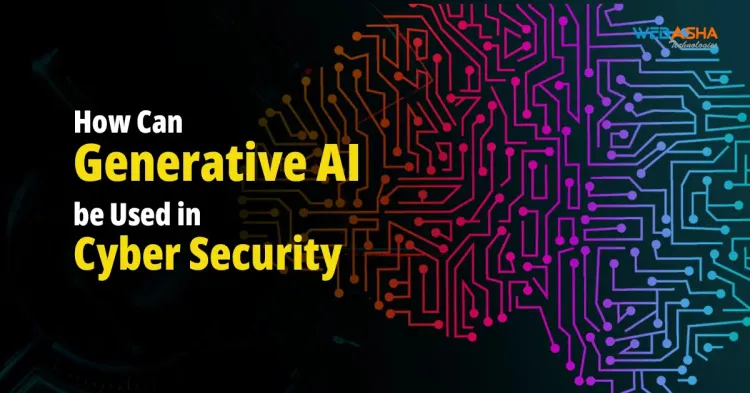
In the rapidly evolving world of cyber security, Generative AI is emerging as a game-changing technology. Its potential to enhance security measures and combat cyber threats is becoming increasingly apparent. This article explores how Generative AI can be utilized in cyber security to create robust defenses, streamline operations, and anticipate potential threats.
How generative AI is improving cybersecurity
1. Enhanced Threat Detection and Response
Generative AI can significantly improve threat detection and response capabilities. Traditional security systems rely on predefined signatures to identify threats. In contrast, Generative AI can analyze vast amounts of data to identify patterns and anomalies that may indicate a security breach. By continuously learning from new data, Generative AI models can detect emerging threats and adapt their detection algorithms in real-time. This dynamic approach enables faster and more accurate identification of potential threats, reducing the window of vulnerability.
2. Automated Threat Simulation and Testing
One of the key applications of Generative AI in cyber security is the automation of threat simulation and testing. Generative AI can create realistic attack scenarios to test the resilience of security systems. By simulating various attack vectors and tactics, security teams can evaluate their defenses and identify vulnerabilities before they are exploited by real attackers. This proactive approach helps organizations strengthen their security posture and improve their incident response strategies.
3. Advanced Phishing Detection
Phishing attacks continue to be a major threat in cyber security. Generative AI can enhance phishing detection by analyzing email content, sender behavior, and other contextual factors. AI models can generate and recognize phishing patterns, distinguishing between legitimate and malicious communications with high accuracy. This capability helps prevent phishing attacks from reaching end-users and reduces the risk of credential theft and data breaches.
4. Improved Malware Analysis
Malware analysis is a critical component of cyber defense. Generative AI can accelerate and enhance the analysis of malicious software by generating synthetic samples and predicting malware behavior. AI models can simulate how malware operates, identify its components, and predict its potential impact. This advanced analysis aids in the development of effective countermeasures and helps security teams stay ahead of evolving threats.
5. Predictive Threat Intelligence
Generative AI can also contribute to predictive threat intelligence. By analyzing historical data and identifying trends, AI models can forecast potential threats and security incidents. This foresight enables organizations to prepare and implement preventative measures before threats materialize. Predictive threat intelligence empowers security teams to make informed decisions and allocate resources more effectively.
6. Optimized Incident Response
Effective incident response is crucial for minimizing the impact of cyber attacks. Generative AI can optimize incident response by automating routine tasks, such as data collection and analysis. AI models can generate actionable insights from incident data, helping security teams prioritize their efforts and respond more efficiently. This automation reduces response times and ensures that critical issues are addressed promptly.
7. Personalized Security Training
Generative AI can also be used to develop personalized security training programs. By analyzing individual user behavior and identifying common vulnerabilities, AI can create tailored training materials and scenarios. This approach enhances user awareness and helps individuals recognize and respond to potential security threats more effectively.
How Generative AI is Threatening Cybersecurity
Generative AI has made significant strides in enhancing various industries, including cybersecurity. However, alongside its benefits, Generative AI also presents new threats that can undermine security measures. This article explores how Generative AI poses challenges to cybersecurity and highlights the potential risks organizations should be aware of.
1. Creation of Sophisticated Phishing Attacks
Generative AI can be used to create highly convincing phishing attacks. By analyzing vast amounts of data from social media and other sources, AI models can generate phishing emails that closely mimic legitimate communications. These AI-generated messages can be tailored to individual targets, making them more likely to deceive recipients and compromise sensitive information.
2. Automated Malware Development
Generative AI can facilitate the automated creation of malware. AI models can generate new strains of malicious software by analyzing existing malware patterns and behaviors. This capability enables cybercriminals to develop sophisticated and evasive malware that can evade traditional security defenses, increasing the risk of data breaches and system infections.
3. Deepfake Technology for Social Engineering
Deepfake technology, powered by Generative AI, poses a significant threat to cybersecurity. By generating realistic but fake audio or video content, cybercriminals can manipulate individuals into divulging confidential information or executing malicious actions. This form of social engineering can be used to impersonate executives, trick employees, or spread misinformation.
4. Adversarial Attacks on AI Models
Generative AI can be used to conduct adversarial attacks on other AI systems. By creating inputs specifically designed to deceive or exploit vulnerabilities in AI models, attackers can compromise the accuracy and effectiveness of security solutions. This can lead to incorrect threat detections, false positives, or missed threats, undermining the reliability of AI-driven security measures.
5. Enhanced Evasion Techniques
Generative AI enables the development of advanced evasion techniques that can bypass traditional security measures. For example, AI-generated code can be used to create polymorphic malware that constantly changes its appearance to avoid detection by antivirus software. These techniques make it more challenging for security systems to identify and neutralize threats.
6. Increased Attack Sophistication
Generative AI can enhance the sophistication of cyber attacks by enabling attackers to devise more complex strategies and tactics. AI models can analyze and learn from previous successful attacks, generating new methods for exploiting vulnerabilities. This increased sophistication makes it more difficult for defenders to anticipate and counteract emerging threats.
7. Manipulation of Security Systems
Generative AI can be used to manipulate security systems by creating fake alerts or misleading data. Attackers can generate false positives or fabricate evidence of security breaches, leading to confusion and misallocation of resources. This can disrupt incident response efforts and hinder the ability of security teams to effectively address real threats.
8. Exploitation of AI-Driven Security Flaws
As organizations adopt AI-driven security solutions, they may inadvertently introduce new vulnerabilities. Generative AI can be used to identify and exploit flaws in these systems. Attackers can generate inputs or scenarios that reveal weaknesses in AI algorithms, potentially compromising the security of the entire system.
Generative AI in Cybersecurity: Examples and Applications
Generative AI is rapidly becoming a crucial component in the field of cybersecurity. By leveraging advanced machine learning techniques, Generative AI enhances various aspects of security, from threat detection to incident response. This article explores several practical examples of how Generative AI is being applied in cybersecurity.
1. AI-Driven Threat Detection
Example: Darktrace's Antigena
Darktrace's Antigena uses Generative AI to identify and respond to threats in real-time. By analyzing network traffic and user behavior, Antigena can generate models of normal and abnormal activities. This allows it to detect anomalies that may indicate a cyber threat, such as unusual data transfers or unexpected login attempts. The system can then take automated actions to mitigate the threat, such as isolating affected devices.
2. Automated Malware Analysis
Example: CylancePROTECT
CylancePROTECT leverages Generative AI to analyze and classify malware. The AI models are trained on vast datasets of known malware samples to identify patterns and generate signatures for new variants. This approach allows CylancePROTECT to detect and block sophisticated malware in real-time, even if it has not been previously encountered.
3. Phishing Detection and Prevention
Example: Mimecast's Targeted Threat Protection
Mimecast's Targeted Threat Protection uses Generative AI to enhance phishing detection. The system analyzes email content and sender behavior, generating models to identify potential phishing attempts. By learning from both known phishing attacks and emerging threats, Mimecast can effectively filter out malicious emails and protect users from phishing scams.
4. Threat Intelligence Generation
Example: Recorded Future
Recorded Future utilizes Generative AI to create predictive threat intelligence. The platform analyzes vast amounts of data from various sources, including dark web forums and social media, to generate insights about potential cyber threats. This predictive capability allows organizations to anticipate and prepare for emerging threats before they materialize.
5. Attack Simulation and Testing
Example: AttackIQ's Security Optimization Platform
AttackIQ uses Generative AI to simulate various attack scenarios and test security defenses. The platform generates realistic attack simulations based on known tactics and techniques used by cybercriminals. By running these simulations, organizations can evaluate their security posture, identify vulnerabilities, and improve their incident response strategies.
6. Deepfake Detection
Example: Sensity AI
Sensity AI employs Generative AI to detect deepfakes and manipulated media. The platform analyzes video and audio content to identify signs of manipulation, such as inconsistencies in facial expressions or voice patterns. This capability helps organizations and individuals recognize and address misinformation and digital forgeries.
7. Personalized Security Training
Example: KnowBe4's Security Awareness Training
KnowBe4 integrates Generative AI into its security awareness training programs. The AI generates personalized training scenarios based on user behavior and common security vulnerabilities. This approach ensures that employees receive targeted training that addresses their specific risk areas, enhancing overall security awareness.
8. Data Protection and Privacy
Example: Privitar
Privitar uses Generative AI to create synthetic data that mimics real datasets without exposing sensitive information. This synthetic data can be used for research and testing purposes while protecting privacy. Additionally, Privitar's AI models analyze data access patterns to detect potential breaches and ensure data security.
What Your Organization Can Do in Response to Generative AI in Cybersecurity
As Generative AI continues to evolve and impact cybersecurity, organizations must proactively address both the opportunities and challenges it presents. From enhancing security measures to mitigating potential risks, here are key actions your organization can take in response to Generative AI in cybersecurity.
1. Invest in AI-Driven Security Solutions
Action: Implement Advanced AI Security Tools
Investing in AI-driven security solutions can significantly enhance your organization’s ability to detect and respond to cyber threats. Advanced tools powered by Generative AI can improve threat detection, automate incident response, and provide predictive threat intelligence. Evaluate and integrate AI-based solutions that align with your security needs to bolster your defenses.
2. Enhance Training and Awareness Programs
Action: Provide Comprehensive AI Security Training
With the rise of Generative AI, it is crucial to ensure that your security team and employees are well-informed about AI-driven threats and technologies. Implement training programs that cover AI-related security risks, such as phishing attacks and deepfakes. Regularly update training materials to reflect new developments in AI and cybersecurity.
3. Adopt a Risk-Based Approach to AI Security
Action: Conduct Risk Assessments and Develop Mitigation Strategies
Perform risk assessments to identify potential vulnerabilities and threats associated with Generative AI. Develop and implement mitigation strategies to address these risks. This includes securing AI systems, monitoring their outputs for anomalies, and establishing protocols for responding to AI-related incidents.
4. Collaborate with AI Experts and Researchers
Action: Engage with AI and Cybersecurity Communities
Collaborate with AI experts and cybersecurity researchers to stay informed about the latest developments and best practices. Participate in industry forums, conferences, and research initiatives to gain insights into emerging threats and innovative solutions. Building strong relationships with experts can help you stay ahead of potential challenges.
5. Strengthen Data Protection Measures
Action: Implement Robust Data Privacy and Security Policies
Generative AI can create synthetic data that mimics real datasets, posing new challenges for data protection. Strengthen your data privacy and security policies to address these challenges. Ensure that data handling practices comply with regulations and implement measures to protect sensitive information from unauthorized access.
6. Monitor and Evaluate AI System Performance
Action: Regularly Assess AI System Effectiveness
Continuously monitor and evaluate the performance of AI systems used in your cybersecurity infrastructure. Regular assessments help ensure that AI tools are functioning as intended and effectively mitigating threats. Adjust and refine AI models based on performance data and emerging threat intelligence.
7. Implement Ethical AI Practices
Action: Establish Guidelines for Responsible AI Use
Adopt ethical AI practices to ensure that AI technologies are used responsibly and transparently. Establish guidelines for the ethical use of AI in cybersecurity, including considerations for bias, fairness, and accountability. Promote a culture of responsible AI use within your organization to maintain trust and integrity.
8. Prepare for AI-Driven Attacks
Action: Develop AI-Resilient Security Strategies
Prepare for the possibility of AI-driven attacks by developing security strategies that address AI-specific threats. This includes implementing defenses against sophisticated phishing attacks, deepfakes, and automated malware. Regularly update your incident response plans to account for AI-related scenarios and threats.
Conclusion
Generative AI holds tremendous promise for enhancing cyber security across various domains. From improving threat detection and response to optimizing incident management and providing personalized training, AI-driven solutions offer significant advantages in combating cyber threats. As technology continues to advance, leveraging Generative AI will become increasingly vital in safeguarding digital assets and ensuring robust security defenses.
FAQs
1. What is Generative AI and how does it relate to cyber security?
Generative AI refers to artificial intelligence systems that can generate new data or content based on learned patterns from existing data. In cyber security, Generative AI is used to create and simulate various attack scenarios, enhance threat detection, automate malware analysis, and improve overall security measures.
2. How does Generative AI improve threat detection?
Generative AI enhances threat detection by analyzing large volumes of data to identify patterns and anomalies that might indicate a security breach. Unlike traditional systems that rely on predefined signatures, Generative AI models continuously learn and adapt, enabling them to detect new and evolving threats more effectively.
3. Can Generative AI help in phishing attack prevention?
Yes, Generative AI can improve phishing attack prevention by analyzing email content, sender behavior, and other contextual factors to detect phishing attempts. AI models can generate and recognize phishing patterns, reducing the likelihood of phishing emails reaching end-users and mitigating the risk of credential theft and data breaches.
4. How does Generative AI assist in malware analysis?
Generative AI assists in malware analysis by generating synthetic samples and predicting malware behavior. By simulating how malware operates and identifying its components, AI models help security teams develop effective countermeasures and stay ahead of evolving threats.
5. What role does Generative AI play in threat intelligence?
Generative AI contributes to predictive threat intelligence by analyzing historical data to identify trends and forecast potential threats. This predictive capability allows organizations to implement preventative measures before threats materialize, enabling proactive defense strategies.
6. How can Generative AI optimize incident response?
Generative AI optimizes incident response by automating routine tasks such as data collection and analysis. AI models generate actionable insights from incident data, helping security teams prioritize and respond to incidents more efficiently, thus reducing response times and minimizing the impact of attacks.
7. Can Generative AI be used for personalized security training?
Yes, Generative AI can be used to develop personalized security training programs. By analyzing individual user behavior and identifying common vulnerabilities, AI can create customized training materials and scenarios, enhancing user awareness and improving their ability to recognize and respond to security threats.
8. Are there any limitations to using Generative AI in cyber security?
While Generative AI offers many benefits, it also has limitations. These include the potential for false positives or negatives, the need for continuous training and updating of models, and the reliance on high-quality data. Additionally, AI systems must be carefully monitored to avoid misuse or unintended consequences.
9. How can organizations start implementing Generative AI in their cyber security strategies?
Organizations can start implementing Generative AI by assessing their current security needs and identifying areas where AI can add value. They should collaborate with AI experts to develop and integrate AI-driven solutions, ensure they have access to high-quality data, and continuously monitor and refine their AI models to maintain effectiveness.
10. What are the future prospects of Generative AI in cyber security?
The future prospects of Generative AI in cyber security are promising. As technology advances, Generative AI is expected to play an increasingly crucial role in detecting and mitigating threats, automating security operations, and providing advanced threat intelligence. Continued innovation and research will likely expand its capabilities and applications in the field.