AI vs. Traditional Fraud Detection Systems | Which Works Better?
As digital transactions increase, businesses need robust fraud detection systems to prevent financial losses. Traditional fraud detection relies on rule-based algorithms, manual reviews, and predefined thresholds, making it less adaptive to evolving fraud tactics. In contrast, AI-powered fraud detection uses machine learning, behavioral analytics, real-time monitoring, and automation to detect and prevent fraudulent activities with greater accuracy and speed. AI reduces false positives, adapts to new fraud techniques, and scales efficiently, making it a superior choice for modern security threats. Businesses in banking, fintech, e-commerce, and digital payments must transition to AI-driven fraud prevention solutions to stay ahead of cybercriminals.
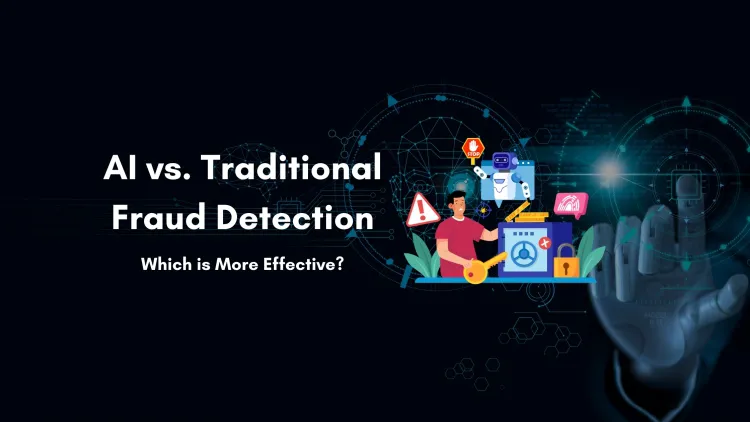
Table of Contents
- Why AI is Essential for Dark Web Monitoring
- How Traditional Fraud Detection Systems Work
- How AI-Powered Fraud Detection Systems Work
- AI vs. Traditional Fraud Detection Systems
- Which Fraud Detection System Works Better?
- Conclusion
- FAQs
Introduction
As digital transactions increase, fraudsters continue to find new ways to exploit security loopholes. To combat fraud, businesses and financial institutions rely on fraud detection systems that analyze transactions and identify suspicious activities. However, the battle between AI-powered fraud detection and traditional rule-based systems raises the question: Which method is more effective?
Artificial Intelligence (AI) has revolutionized fraud detection by leveraging machine learning, behavioral analysis, and automation to detect fraudulent activities in real time. In contrast, traditional fraud detection systems rely on pre-set rules, manual reviews, and historical data analysis to identify threats. This blog will compare AI and traditional fraud detection systems, their effectiveness, challenges, and which one works best for modern security threats.
How Traditional Fraud Detection Systems Work
Traditional fraud detection systems operate on rule-based algorithms and manual analysis. They identify fraud based on predefined conditions and flag transactions that violate set rules.
Key Features of Traditional Fraud Detection
- Rule-Based Approach: Uses "if-then" rules to detect fraud, such as blocking transactions from high-risk locations.
- Manual Review Processes: Transactions flagged as suspicious are manually reviewed by analysts.
- Static Thresholds: Limits are set for transaction amounts, login attempts, or frequency of transactions.
- Blacklist and Whitelist Systems: Pre-approved users are allowed, while suspicious users are blocked.
- Pattern Matching: Recognizes known fraudulent patterns based on historical data.
Limitations of Traditional Fraud Detection
- High False Positives: Many legitimate transactions are mistakenly flagged, frustrating customers.
- Inability to Detect New Fraud Techniques: New fraud methods are often undetectable by static rule-based systems.
- Scalability Issues: As transactions increase, manual review processes become inefficient.
- Slow Detection and Response: Traditional methods require human intervention, leading to delays in fraud prevention.
How AI-Powered Fraud Detection Systems Work
AI-driven fraud detection uses machine learning (ML), deep learning, and real-time data analysis to detect fraudulent behavior. Unlike traditional systems, AI adapts to new fraud techniques by learning from historical and real-time transaction data.
Key Features of AI-Powered Fraud Detection
- Machine Learning Algorithms: AI learns from past fraud patterns and continuously improves detection accuracy.
- Behavioral Analysis: AI monitors user behavior (e.g., transaction frequency, device usage) to detect anomalies.
- Real-Time Fraud Detection: AI processes data instantly, identifying fraud as it happens.
- Adaptive Learning: AI updates fraud detection models dynamically to detect evolving fraud techniques.
- Automated Decision-Making: Reduces reliance on manual reviews, allowing faster fraud mitigation.
Advantages of AI-Powered Fraud Detection
- Higher Accuracy: AI reduces false positives by distinguishing legitimate transactions from fraudulent ones.
- Detects Unknown Fraud Techniques: AI can identify new, emerging fraud patterns without needing predefined rules.
- Scalability: AI efficiently handles large transaction volumes without human intervention.
- Reduced Manual Work: Automation minimizes the need for analysts to review every transaction.
- Real-Time Prevention: AI flags and blocks fraudulent activities instantly.
AI vs. Traditional Fraud Detection Systems
Feature | Traditional Fraud Detection | AI-Powered Fraud Detection |
---|---|---|
Detection Approach | Rule-based, static | Machine learning, adaptive |
Accuracy | High false positives | Low false positives |
Fraud Adaptation | Cannot detect new fraud techniques | Learns and adapts to evolving fraud |
Speed | Manual reviews slow detection | Real-time fraud detection |
Scalability | Struggles with high transaction volumes | Easily scalable |
Automation Level | Requires human intervention | Fully automated fraud detection |
Behavioral Analysis | Not included | Analyzes user behavior patterns |
Real-Time Alerts | Delayed alerts | Instant fraud detection |
Which Fraud Detection System Works Better?
When to Use Traditional Fraud Detection
Traditional fraud detection may still be useful for small businesses or low-risk environments where fraud rates are minimal. Rule-based systems are effective when fraud patterns are well-known and static. However, they are not ideal for businesses with high transaction volumes or evolving fraud threats.
When to Use AI-Powered Fraud Detection
For organizations dealing with large-scale transactions, online banking, e-commerce, and digital payments, AI-powered fraud detection is the best choice. AI's ability to detect complex fraud schemes, analyze behavior, and provide real-time responses makes it superior to traditional systems.
Future of Fraud Detection: AI is the Future
AI-powered fraud detection is becoming the industry standard due to its efficiency, adaptability, and ability to handle evolving fraud threats. Businesses investing in AI-driven fraud prevention tools will stay ahead of cybercriminals and reduce financial losses.
Conclusion
While traditional fraud detection relies on predefined rules and manual reviews, AI-powered fraud detection offers real-time threat detection, automated decision-making, and adaptive learning. AI is the superior choice for modern fraud prevention as it detects fraud faster, more accurately, and with minimal human intervention.
As cybercriminals develop sophisticated fraud techniques, businesses must upgrade from traditional rule-based systems to AI-driven fraud detection solutions. The future of fraud prevention lies in machine learning, predictive analytics, and real-time fraud detection, ensuring secure online transactions and customer trust.
FAQs
How do traditional fraud detection systems work?
Traditional fraud detection systems use predefined rules, blacklists, manual reviews, and pattern matching to identify fraudulent activities. They rely on historical fraud data to detect suspicious transactions.
What are the limitations of traditional fraud detection methods?
Traditional systems struggle with false positives, slow detection times, inability to detect new fraud patterns, high operational costs, and poor scalability as fraud tactics evolve.
How does AI improve fraud detection compared to traditional methods?
AI-powered fraud detection uses machine learning, real-time data processing, and behavioral analysis to detect fraud patterns dynamically, reducing false positives and improving detection speed.
What role does machine learning play in fraud detection?
Machine learning models analyze past fraud data, user behavior, and transaction anomalies to detect emerging fraud techniques without relying on static rules.
How does AI detect new fraud techniques?
AI continuously learns from real-time transaction data, identifying anomalies and predicting fraudulent behavior without requiring manual rule updates.
What is behavioral analysis in AI fraud detection?
Behavioral analysis monitors user habits, typing speed, login locations, and transaction frequency to detect unusual patterns that indicate fraud.
Does AI-powered fraud detection prevent false positives?
Yes, AI significantly reduces false positives by distinguishing between legitimate and fraudulent activities using risk-based authentication and pattern recognition.
Can AI replace human analysts in fraud detection?
AI automates fraud detection but still requires human oversight for complex cases and decision-making in edge scenarios.
How does AI detect account takeovers?
AI flags unusual login attempts, IP address changes, and device mismatches to detect account takeovers (ATO) and prevent unauthorized access.
What is real-time fraud detection, and why is it important?
Real-time fraud detection uses instant transaction analysis to block fraud as it happens, preventing financial losses before they occur.
Can AI handle large transaction volumes?
Yes, AI-driven systems can process millions of transactions per second, making them highly scalable for banks, fintech companies, and e-commerce platforms.
How does AI fraud detection benefit e-commerce businesses?
AI helps e-commerce platforms detect fake accounts, payment fraud, chargeback fraud, and bot-driven attacks, improving security and customer trust.
How does AI-powered fraud detection enhance online banking security?
AI secures online banking by monitoring customer behavior, flagging high-risk transactions, and detecting phishing or malware attacks in real-time.
What is risk-based authentication in fraud detection?
Risk-based authentication (RBA) assigns risk scores to transactions, requiring additional verification for high-risk users while allowing seamless access for legitimate ones.
How does AI detect money laundering in financial transactions?
AI detects suspicious money transfers, unusual cash deposits, and structuring patterns that indicate money laundering activities.
What industries benefit from AI fraud detection?
Industries such as banking, fintech, insurance, healthcare, gaming, travel, and e-commerce use AI to prevent fraud and enhance security.
How does AI help detect credit card fraud?
AI analyzes spending patterns, location mismatches, and unusual purchase behavior to detect and block fraudulent credit card transactions.
What is device fingerprinting in AI fraud detection?
Device fingerprinting tracks browser settings, device type, and network configurations to identify fraudsters using stolen credentials.
Does AI-powered fraud detection require customer data?
Yes, AI relies on transactional data, biometric authentication, and behavioral insights while ensuring compliance with data privacy regulations like GDPR and CCPA.
Can AI detect insider fraud?
AI detects insider fraud by monitoring employee behavior, flagging suspicious access requests, unauthorized transactions, and policy violations.
How does AI help businesses comply with fraud regulations?
AI ensures compliance with AML (Anti-Money Laundering), KYC (Know Your Customer), PSD2, and PCI DSS regulations by automating identity verification and fraud monitoring.
How does AI prevent phishing attacks?
AI detects phishing emails, fake login pages, and malicious websites using natural language processing (NLP) and anomaly detection.
What is adaptive fraud prevention?
Adaptive fraud prevention allows AI to dynamically adjust fraud detection models based on real-time threat intelligence and evolving attack patterns.
Can AI-powered fraud detection be integrated with legacy systems?
Yes, AI solutions offer API-based integrations to work alongside existing fraud detection tools and legacy security infrastructure.
How does AI prevent payment fraud in digital wallets?
AI secures digital wallets by verifying biometric authentication, monitoring transaction history, and detecting unauthorized payments.
Does AI-powered fraud detection work for small businesses?
Yes, AI-driven fraud prevention tools are available for small businesses and startups, offering cost-effective real-time fraud detection and risk management.
How does AI handle fraudulent refund claims?
AI detects refund fraud by analyzing customer refund patterns, purchase history, and IP address mismatches to prevent chargeback abuse.
How does AI combat synthetic identity fraud?
AI cross-references identity documents, biometric data, and digital footprints to detect synthetic identities created using stolen or fake credentials.
What are the biggest challenges of AI-powered fraud detection?
Challenges include deepfake fraud, algorithm bias, regulatory compliance, data privacy concerns, and integration complexity with legacy systems.