AI vs. Human Analysts in Fraud Detection | Which One is More Effective for Preventing Financial Crimes, Cyber Fraud, and Identity Theft?
Fraud detection is evolving with the rise of Artificial Intelligence (AI) and human analysts working together to combat financial fraud, cybercrime, identity theft, and money laundering. AI-powered fraud detection systems can analyze millions of transactions in real-time, identifying fraudulent patterns, phishing attempts, and account takeovers. Meanwhile, human analysts bring critical thinking, intuition, and investigative skills to detect complex fraud cases that AI might miss. While AI is faster, scalable, and data-driven, it sometimes generates false positives and struggles with social engineering and deepfake frauds. On the other hand, human analysts excel in contextual understanding, compliance, and fraud investigations but face limitations in handling vast amounts of data. The best fraud detection strategy is a hybrid AI-human approach, combining AI automation with human expertise for maximum accuracy and efficiency.
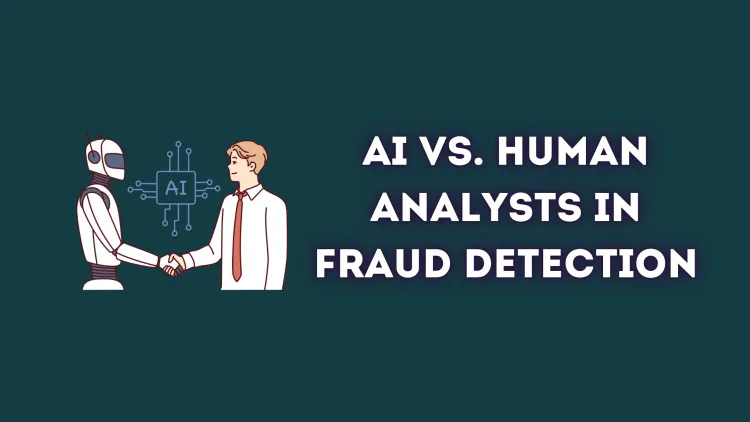
Table of Contents
- Introduction
- How AI Detects Fraud
- How Human Analysts Detect Fraud
- AI vs. Human Analysts: Pros and Cons
- Which Is Better: AI, Human Analysts, or a Hybrid Approach?
Introduction
Financial fraud is a growing global challenge, with businesses and individuals losing billions of dollars annually due to cybercrime, identity theft, money laundering, and phishing scams. To combat this, organizations rely on two primary fraud detection methods: Artificial Intelligence (AI) and human analysts.
But the big question remains: Who detects fraud better—AI or human experts?
AI offers speed, automation, and data-driven insights, while human analysts provide intuition, contextual understanding, and experience. In this blog, we will explore:
✔ How AI-powered fraud detection works
✔ The role of human analysts in fraud investigation
✔ Pros and cons of AI vs. human fraud detection
✔ Which is more effective: AI, human experts, or a combination of both?
How AI Detects Fraud
AI-powered fraud detection uses machine learning, deep learning, and data analytics to analyze large volumes of transactions and identify suspicious patterns. AI systems are trained using historical fraud data and can adapt to new threats.
1. AI for Real-Time Fraud Detection
✔ AI analyzes millions of transactions per second to detect fraud instantly.
✔ Identifies unusual spending patterns, account takeovers, and fake identities.
✔ Flags high-risk transactions for manual review by human analysts.
2. Machine Learning (ML) for Anomaly Detection
✔ ML algorithms detect deviations from normal transaction behaviors.
✔ Identifies new types of fraud even without pre-defined rules.
✔ Continuously improves accuracy based on past fraud cases.
3. AI-Powered Behavioral Analytics
✔ AI monitors user behavior, login locations, and transaction histories.
✔ Detects identity theft, synthetic fraud, and account takeovers.
✔ Prevents fraud by verifying users based on their typical habits.
4. AI in Phishing & Social Engineering Attack Prevention
✔ Uses Natural Language Processing (NLP) to detect fake emails, messages, and scam calls.
✔ Identifies suspicious communication patterns and fraudulent requests.
✔ Blocks phishing attempts before they reach users.
5. AI in Biometric Authentication & Risk-Based Security
✔ AI enhances security using facial recognition, fingerprint scanning, and voice authentication.
✔ Blocks access to accounts with suspicious login behaviors.
✔ Uses risk-based authentication for high-value transactions.
How Human Analysts Detect Fraud
While AI automates fraud detection, human experts bring critical thinking, intuition, and investigative skills to uncover complex fraud schemes.
1. Manual Fraud Review & Investigation
✔ Analysts review flagged transactions to determine if fraud occurred.
✔ They analyze context, intent, and past fraud reports.
✔ Apply reasoning to avoid false positives and legitimate transaction blocks.
2. Understanding Social Engineering & Psychological Tactics
✔ Fraudsters use manipulation, impersonation, and deception to commit financial crimes.
✔ Human analysts recognize patterns that AI may miss, such as fraudulent narratives or emotional tactics.
3. Fraud Pattern Recognition & Threat Intelligence
✔ Analysts use experience to connect different fraud cases and identify trends.
✔ They analyze dark web data, social media, and hacker forums to predict future threats.
4. Legal & Compliance Considerations
✔ Analysts ensure fraud detection aligns with regulatory requirements and legal standards.
✔ They handle disputes, chargebacks, and fraud claims with human judgment.
AI vs. Human Analysts: Pros and Cons
Feature | AI Fraud Detection | Human Analyst Fraud Detection |
---|---|---|
Speed | Detects fraud in milliseconds | Takes minutes to hours per case |
Scalability | Analyzes millions of transactions instantly | Limited by human workload |
Accuracy | High for structured data and patterns | Better for contextual fraud and social engineering |
Adaptability | Learns from historical fraud cases | Adapts based on new fraud schemes |
False Positives | Sometimes flags legitimate transactions as fraud | Uses judgment to reduce false positives |
Understanding Complex Fraud | Struggles with emotional manipulation & social engineering | Understands intent, deception, and fraud networks |
Legal & Compliance | Ensures automatic rule-based compliance | Applies human judgment for legal concerns |
Cost | Lower long-term costs after initial AI setup | Higher costs due to salaries & training |
Which Is Better: AI, Human Analysts, or a Hybrid Approach?
1. AI-Only Fraud Detection: Strengths & Weaknesses
✔ Pros: Faster, scalable, and data-driven.
❌ Cons: May generate false positives and struggle with complex fraud cases.
2. Human-Only Fraud Detection: Strengths & Weaknesses
✔ Pros: Better at social engineering detection, contextual analysis, and dispute resolution.
❌ Cons: Slow, expensive, and cannot analyze millions of transactions manually.